-
Notifications
You must be signed in to change notification settings - Fork 177
Commit
This commit does not belong to any branch on this repository, and may belong to a fork outside of the repository.
Merge branch 'master' into bugix/fix-pyfiglet-version-error-#1773
- Loading branch information
Showing
182 changed files
with
16,168 additions
and
1,791 deletions.
There are no files selected for viewing
This file contains bidirectional Unicode text that may be interpreted or compiled differently than what appears below. To review, open the file in an editor that reveals hidden Unicode characters.
Learn more about bidirectional Unicode characters
Original file line number | Diff line number | Diff line change |
---|---|---|
@@ -0,0 +1,46 @@ | ||
name: Build Docker image | ||
on: | ||
workflow_dispatch: | ||
|
||
env: | ||
REGISTRY: ghcr.io | ||
IMAGE_NAME: ${{ github.repository }} | ||
|
||
jobs: | ||
build-and-push-docker-image: | ||
runs-on: ubuntu-latest | ||
permissions: | ||
contents: read | ||
packages: write | ||
|
||
steps: | ||
- name: Checkout Elementary | ||
uses: actions/checkout@v4 | ||
|
||
- name: Set up QEMU for multi-platform support | ||
uses: docker/setup-qemu-action@v3 | ||
|
||
- name: Set up Docker Buildx for multi-platform support | ||
uses: docker/setup-buildx-action@v3 | ||
|
||
- name: Log in to the container registry | ||
uses: docker/login-action@v2 | ||
with: | ||
registry: ${{ env.REGISTRY }} | ||
username: ${{ github.actor }} | ||
password: ${{ secrets.GITHUB_TOKEN }} | ||
|
||
- name: Extract metadata (tags, labels) for Docker | ||
id: meta | ||
uses: docker/metadata-action@v4 | ||
with: | ||
images: ${{ env.REGISTRY }}/${{ env.IMAGE_NAME }} | ||
|
||
- name: Build and push Docker image | ||
uses: docker/build-push-action@v3 | ||
with: | ||
context: . | ||
push: false | ||
platforms: linux/amd64,linux/arm64 | ||
tags: ${{ steps.meta.outputs.tags }} | ||
labels: ${{ steps.meta.outputs.labels }} |
This file contains bidirectional Unicode text that may be interpreted or compiled differently than what appears below. To review, open the file in an editor that reveals hidden Unicode characters.
Learn more about bidirectional Unicode characters
This file contains bidirectional Unicode text that may be interpreted or compiled differently than what appears below. To review, open the file in an editor that reveals hidden Unicode characters.
Learn more about bidirectional Unicode characters
Original file line number | Diff line number | Diff line change |
---|---|---|
|
@@ -16,6 +16,5 @@ types-pytz | |
types-jsonschema | ||
types-PyYAML | ||
types-setuptools | ||
pandas-stubs | ||
types-retry | ||
types-decorator |
This file contains bidirectional Unicode text that may be interpreted or compiled differently than what appears below. To review, open the file in an editor that reveals hidden Unicode characters.
Learn more about bidirectional Unicode characters
This file contains bidirectional Unicode text that may be interpreted or compiled differently than what appears below. To review, open the file in an editor that reveals hidden Unicode characters.
Learn more about bidirectional Unicode characters
This file contains bidirectional Unicode text that may be interpreted or compiled differently than what appears below. To review, open the file in an editor that reveals hidden Unicode characters.
Learn more about bidirectional Unicode characters
Original file line number | Diff line number | Diff line change |
---|---|---|
@@ -0,0 +1,150 @@ | ||
--- | ||
title: "Detection and coverage" | ||
--- | ||
|
||
In Elementary you can detect data issues by combining data validations (as dbt tests, custom SQL) and anomaly detection monitors. | ||
|
||
As you expand your coverage, it's crucial to balance between coverage and meaningful detections. While it may seem attractive to implement extensive monitoring throughout your data infrastructure, this approach is often suboptimal. Excessive failures can lead to alerts fatigue, potentially causing them to overlook significant issues. Additionally, such approach will incur unnecessary compute costs. | ||
|
||
In this section we will cover the available tests in Elementary, recommended tests for common use cases, and how to use the data quality dimensions framework to improve coverage. | ||
|
||
## Supported data tests and monitors | ||
|
||
Elementary detection includes: | ||
|
||
- Data tests - Validate an explicit expectation, and fail if it is not met. | ||
- Example: validate there are no null values in a column. | ||
- Anomaly detection monitors - Track a data quality metric over time, and fail if there is an anomaly comparing to previous values and trend. | ||
- Example: track the rate of null values in a column over time, fail if there is a spike. | ||
|
||
### Data tests - | ||
|
||
- dbt tests - Built in dbt tests (`not_null`, `unique`, `accepted_values`, `relationship` ) | ||
- dbt packages - Any dbt package test, we recommend installing `dbt-utils` and `dbt-expectations` . | ||
- Custom SQL tests - Custom query, will pass if no results and fail if any results are returned. | ||
|
||
### Anomaly detection monitors - | ||
|
||
Elementary offers two types of anomaly detection monitors: | ||
|
||
- **Automated Monitors** - Out-of-the-box volume and freshness monitors activated automatically, that query metadata only. | ||
- **Opt-in anomaly detection tests** - Monitors that query raw data and require configuration. | ||
|
||
<Check> | ||
### Recommendations | ||
|
||
- Deploy the packages dbt-utils and dbt-expectations in your dbt projects, to enrich your available tests | ||
- Refer to the [dbt test hub](https://www.elementary-data.com/dbt-test-hub) by Elementary, to explore available tests by use case | ||
</Check> | ||
|
||
## Fine-tuning automated monitors | ||
|
||
As soon as you connect Elementary Cloud Platform to your data warehouse, a backfill process will begin to collect historical metadata. Within an average of a few hours, your automated monitors will be operational. By default, Elementary collects at least 21 days of historical metadata. | ||
|
||
You can fine tune the [**configuration**](https://docs.elementary-data.com/features/anomaly-detection/monitors-configuration) and [**provide feedback**](https://docs.elementary-data.com/features/anomaly-detection/monitors-feedback) to adjust the detection to your needs. | ||
|
||
You can read here about how to interpret the result, and what are the available setting of each monitor: | ||
|
||
- [Automated Freshness](https://docs.elementary-data.com/features/anomaly-detection/automated-freshness) | ||
- [Automated Volume](https://docs.elementary-data.com/features/anomaly-detection/automated-volume) | ||
|
||
## Common testing use cases | ||
|
||
We have the following recommendations for testing different data assets: | ||
|
||
### Data sources | ||
|
||
To detect issues in sources updates, you should monitor volume, freshness and schema: | ||
|
||
- Volume and freshness | ||
- Data updates - Elementary cloud provides automated monitors for freshness and volume. **These are metadata monitors.** | ||
- Updates freshness vs. data freshness - The automated freshness will detect delays in **updates**. \*\*\*\*However, sometimes the update will be on time, but the data itself will be outdated. | ||
- Data freshness (advanced) - Sometimes a table can update on time, but the data itself will be outdated. If you want to validate the freshness of the raw data by relaying on the actual timestamp, you can use: | ||
- Explicit threshold [freshness dbt tests](https://www.elementary-data.com/dbt-test-hub) such as `dbt_utils.recency` , or [dbt source freshness](https://docs.getdbt.com/docs/deploy/source-freshness). | ||
- Elementary `event_freshness_anomalies` to detect anomalies. | ||
- Data volume (advanced) - Although a table can be updated as expected, the data itself might still be imbalanced in terms of volume per specific segment. There are several tests available to monitor that: | ||
- Explicit [volume expectations](https://www.elementary-data.com/dbt-test-hub) such as `expect_table_row_count_to_be_between`. | ||
- Elementary `dimension_anomalies` , that will count rows grouped by a column or combination of columns and can detect drops or spikes in volume in specific subsets of the data. | ||
- Schema changes | ||
|
||
- Automated schema monitors are coming soon: | ||
- These monitors will detect breaking changes to the schema only for columns being consumed based on lineage. | ||
- For now, we recommend defining schema tests on the sources consumed by downstream staging models. | ||
|
||
Some validations on the data itself should be added in the source tables, to test early in the pipeline and detect when data is arriving with an issue from the source. | ||
|
||
- Low cardinality columns / strict set of values - If there are fields with a specific set of values you expect use `accepted_values`. If you also expect a consistency in ratio of these values, use `dimension_anomalies` and group by this column. | ||
- Business requirements - If you are aware of expectations specific to your business, try to enforce early to detect when issues are at the source. Some examples: `expect_column_values_to_be_between`, `expect_column_values_to_be_increasing`, `expect-column-values-to-have-consistent-casing` | ||
|
||
<Check> | ||
### Recommendations | ||
|
||
- Add data freshness and volume validations for relevant source tables, on top of the automated monitors (advanced) | ||
- Add schema tests for source tables | ||
</Check> | ||
|
||
### Primary / foreign key columns in your transformation models | ||
|
||
Tables should be covered with: | ||
|
||
- Unique checks on primary / foreign key columns to detect unnecessary duplications during data transformations. | ||
- Not null checks on primary / foreign key columns to detect missing values during data transformations. | ||
|
||
For incremental tables, it’s recommended to use a `where` clause in the tests, and only validate recent data. This will prevent running the tests on large data sets which is costly and slow. | ||
|
||
<Check> | ||
#### Recommendations | ||
|
||
- Add `unique` and `not_null` tests to key columns | ||
</Check> | ||
|
||
### Public tables | ||
|
||
As these are your data products, coverage here is highly important. | ||
|
||
- Consistency with sources (based on aggregation/primary keys) | ||
- Volume and freshness | ||
- Unique and not null checks on primary keys | ||
- Schema to ensure the "API" to data consumers is not broken | ||
- Business Metrics / KPIs | ||
- Sum / max anomalies group by your critical dimensions / segments (For example - country, platform…) | ||
|
||
### Data quality dimensions framework | ||
|
||
To ensure your detection and coverage have a solid baseline, we recommend leveraging the quality dimensions framework for your critical and public assets. | ||
|
||
The quality dimensions framework divides data validation into six common dimensions: | ||
|
||
- **Completeness**: No missing values, empty values, nulls, etc. | ||
- **Uniqueness**: The data is unique, with no duplicates. | ||
- **Freshness**: The data is up to date and within the expected SLAs. | ||
- **Validity**: The data is in the correct format and structure. | ||
- **Accuracy**: The data adheres to our business requirements and constraints. | ||
- **Consistency**: The data is consistent from sources to targets, and from sources to where it is consumed. | ||
|
||
Elementary has already categorized all the existing tests in the dbt ecosystem, including all elementary anomaly detection monitors, into these quality dimensions and provides health scores per dimension automatically. It also shows if there are coverage gaps per dimension. | ||
|
||
We highly recommend going to the relevant quality dimension, then filtering by a business domain tag to see your coverage gaps in that domain. | ||
|
||
Example - | ||
|
||
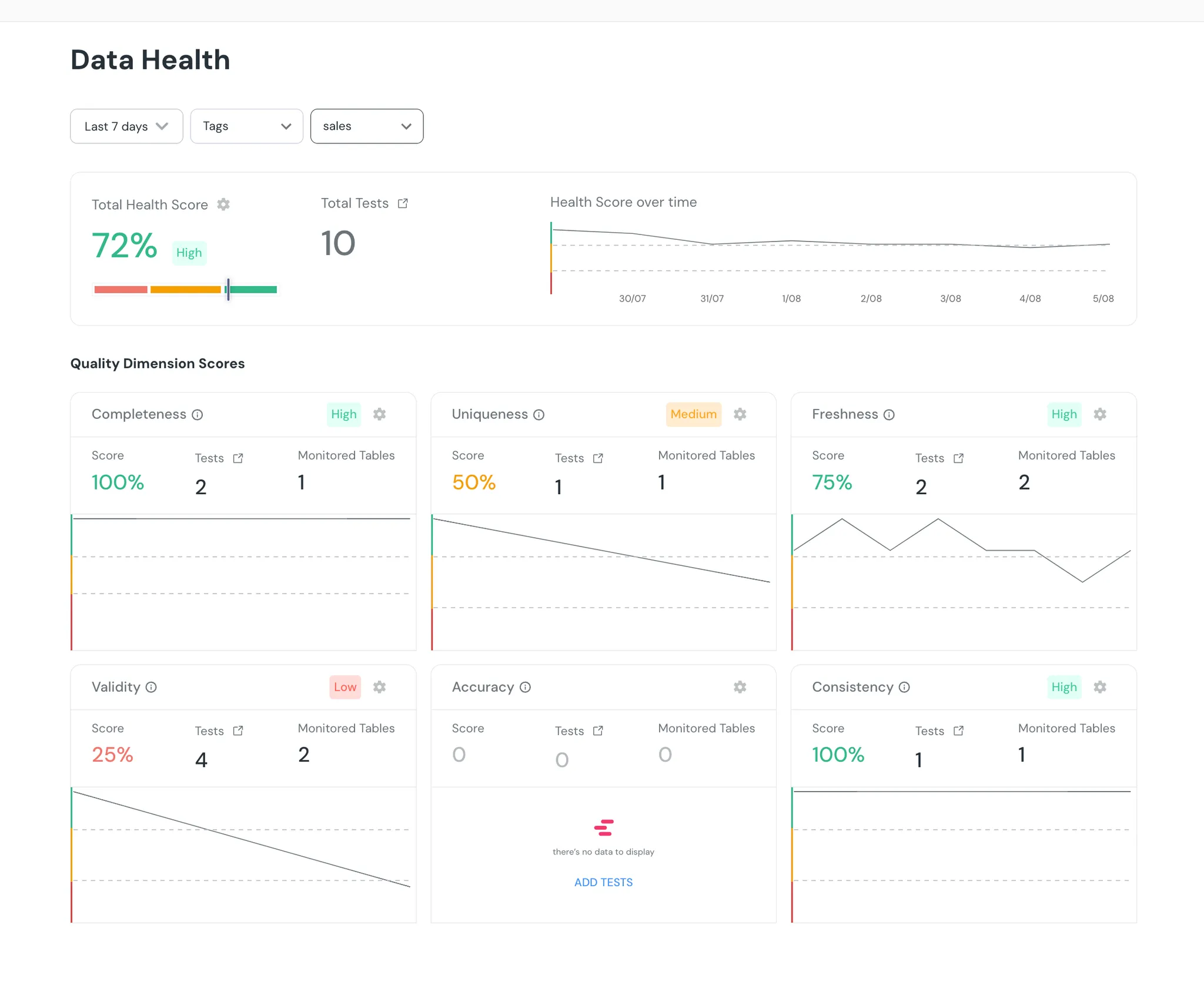 | ||
|
||
In this example, you can see that accuracy tests are missing for our sales domain. This means we don't know if the data in our public-facing "sales" tables adheres to our business constraints. For example, if we have an e-commerce shop where no product has a price below $100 or above $1000, we can easily add a test to validate this. Implementing validations for the main constraints in this domain will allow us to get a quality score for the accuracy level of our data. | ||
|
||
NOTE: The `Test Coverage` page in Elementary allows adding any dbt test from the ecosystem, Elementary anomaly detection monitors, and custom SQL tests. We are working on making it easier to add tests by creating a test catalog organized by quality dimensions and common use cases. | ||
|
||
Example for tests in each quality dimension - | ||
|
||
- **Completeness**: | ||
- not_null, null count, null percent, missing values, empty values, column anomalies on null count, null percent, etc | ||
- **Uniqueness**: | ||
- unique, expect_column_values_to_be_unique, expect_column_unique_value_count_to_be_between, expect_compound_columns_to_be_unique | ||
- **Freshness**: The data is up to date and within the expected SLAs. | ||
- Elementary automated freshness monitor, dbt source freshness, dbt_utils.recency, expect_grouped_row_values_to_have_recent_data | ||
- **Validity**: The data is in the correct format and structure. | ||
- expect_column_values_to_match_regex, expect_column_min_to_be_between, expect_column_max_to_be_between, expect_column_value_lengths_to_be_between, column anomalies on min, max, string lengths | ||
- **Accuracy**: The data adheres to our business requirements and constraints. | ||
- expression_is_true, custom SQL | ||
- **Consistency**: The data is consistent from sources to targets, and from sources to where it is consumed. | ||
- relationship, expect_table_row_count_to_equal_other_table, expect_table_aggregation_to_equal_other_table |
Oops, something went wrong.