-
Notifications
You must be signed in to change notification settings - Fork 6
New issue
Have a question about this project? Sign up for a free GitHub account to open an issue and contact its maintainers and the community.
By clicking “Sign up for GitHub”, you agree to our terms of service and privacy statement. We’ll occasionally send you account related emails.
Already on GitHub? Sign in to your account
Bad results of generating images of KITTI dataset #10
Comments
Hi @withbrightmoon , thank you for the interest in our work. I'll try my best to help you out. It definitely looks like the deep feature statistics loss This is how we can go about debugging the image generation process:
Can you try step (1) post the results? My guess is that without |
Hi @akshaychawla, thanks for your kind reply. I have conducted some experiments, here are some preliminary experimental results. In order to simplify the problem, I only keep one bounding box label for each image. The batch size is set to 16.
Thank you for your detailed reply and attention! Best, |
Thanks for running these experiments Xiu. We can atleast see that the images are being optimized w.r.t the losses that are enabled. The dark images in experiment 2 show that total variation loss is working.
Once you can confirm that you can see some good features, then it makes sense to turn on data augmentation methods to improve performance. Looking forward to your results! |
Sorry for the late reply. I was busy with another project about action recognition this week and did not find time to do experiments. Next week, I will carry out some experiments in accordance with your instructions and report the results. Thank you very much for your detailed reply. Happy New Year! Best, |
Hi @akshaychawla , thanks for your kind guidance, sorry for the late reply. I did some experiments in accordance with your instructions, and got better results. The following is the record of some experiments. For simplicity, the bounding box is set to 80*80 in the center.
Thanks again for your help! Best, |
Hi @withbrightmoon , Thank you for running these experiments, the results look objectively better than before. I apologize for not responding earlier. Here are a few more things that you can try to improve the quality of images:
I’m trying to remember a few ideas to improve image diversity and will update with more ideas. |
it happened in ours experiments too, my arch is retinanet, the generate image close to noise |
@withbrightmoon Hi, I am really interested in this work based on the KITTI dataset. Could you share your code with me? My email address is liuhe_work@126.com. Looking forward to your reply. Thanks a lot! |
Hi @akshaychawla. Thanks for the code.
I tried to generate images of KITTI dataset with yolov3 model but got bad results. I used my own yolov3 pretrained model / cfg file and KITTI dataset. From the 'losses.log' file I found the parameter 'unweighted/loss_r_feature' was 1083850.375. After changing the parameter 'self.bn_reg_scale' to 0.00001, the results are also bad.
I am not sure if there is a problem with my use of the code and also confused about why the parameter 'unweighted/loss_r_feature' is so big. Could you give me some guidance?
Best,
Xiu
1.Results of 2500 iteration:
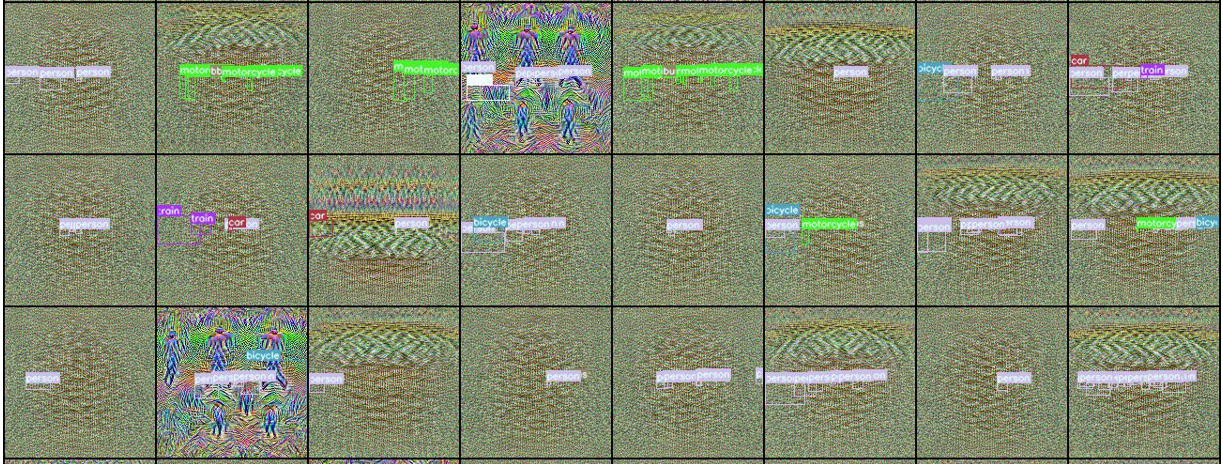
2.losses.log of 1/2500 iteration:
ITERATION: 1
weighted/total_loss 108692.2578125
weighted/task_loss 174.9200897216797
weighted/prior_loss_var_l1 117.44781494140625
weighted/prior_loss_var_l2 0.0
weighted/loss_r_feature 108385.0390625
weighted/loss_r_feature_first 14.853784561157227
unweighted/task_loss 349.8401794433594
unweighted/prior_loss_var_l1 1.5659708976745605
unweighted/prior_loss_var_l2 6894.822265625
unweighted/loss_r_feature 1083850.375
unweighted/loss_r_feature_first 7.426892280578613
unweighted/inputs_norm 12.4415922164917
learning_Rate 0.1999999210431752
ITERATION: 2500
weighted/total_loss 58120.15625
weighted/task_loss 101.14430236816406
weighted/prior_loss_var_l1 77.38021850585938
weighted/prior_loss_var_l2 0.0
weighted/loss_r_feature 57935.38671875
weighted/loss_r_feature_first 6.245403289794922
unweighted/task_loss 202.28860473632812
unweighted/prior_loss_var_l1 1.0317362546920776
unweighted/prior_loss_var_l2 4149.73193359375
unweighted/loss_r_feature 579353.875
unweighted/loss_r_feature_first 3.122701644897461
unweighted/inputs_norm 13.469326972961426
learning_Rate 0.0
Verifier InvImage mPrec: 0.005173 mRec: 0.001166 mAP: 0.0006404 mF1: 0.001902
Teacher InvImage mPrec: 0.005173 mRec: 0.001166 mAP: 0.0006404 mF1: 0.001902
Verifier GeneratedImage mPrec: 0.005173 mRec: 0.001166 mAP: 0.0006404 mF1: 0.001902
tensor(7.42703, device='cuda:0', grad_fn=)
tensor(12243.45508, device='cuda:0', grad_fn=)
tensor(696.13055, device='cuda:0', grad_fn=)
tensor(3364.34961, device='cuda:0', grad_fn=)
tensor(23411.76953, device='cuda:0', grad_fn=)
tensor(1157.99390, device='cuda:0', grad_fn=)
tensor(10253.75781, device='cuda:0', grad_fn=)
tensor(805.68719, device='cuda:0', grad_fn=)
tensor(2327.99268, device='cuda:0', grad_fn=)
tensor(28308.19727, device='cuda:0', grad_fn=)
tensor(875.56348, device='cuda:0', grad_fn=)
tensor(2283.58887, device='cuda:0', grad_fn=)
tensor(986.32434, device='cuda:0', grad_fn=)
tensor(16160.01953, device='cuda:0', grad_fn=)
tensor(1146.45435, device='cuda:0', grad_fn=)
tensor(2227.72607, device='cuda:0', grad_fn=)
tensor(891.68048, device='cuda:0', grad_fn=)
tensor(1558.72815, device='cuda:0', grad_fn=)
tensor(976.82690, device='cuda:0', grad_fn=)
tensor(1683.61230, device='cuda:0', grad_fn=)
tensor(942.91931, device='cuda:0', grad_fn=)
tensor(770.93372, device='cuda:0', grad_fn=)
tensor(981.38751, device='cuda:0', grad_fn=)
tensor(775.02832, device='cuda:0', grad_fn=)
tensor(875.90454, device='cuda:0', grad_fn=)
tensor(673.36096, device='cuda:0', grad_fn=)
tensor(24172.25781, device='cuda:0', grad_fn=)
tensor(773.39252, device='cuda:0', grad_fn=)
tensor(23998.14844, device='cuda:0', grad_fn=)
tensor(705.16992, device='cuda:0', grad_fn=)
tensor(7424.77148, device='cuda:0', grad_fn=)
tensor(928.11621, device='cuda:0', grad_fn=)
tensor(3338.66113, device='cuda:0', grad_fn=)
tensor(896.17908, device='cuda:0', grad_fn=)
tensor(2490.50635, device='cuda:0', grad_fn=)
tensor(788.92633, device='cuda:0', grad_fn=)
tensor(2501.64746, device='cuda:0', grad_fn=)
tensor(872.77161, device='cuda:0', grad_fn=)
tensor(1576.98535, device='cuda:0', grad_fn=)
tensor(738.18060, device='cuda:0', grad_fn=)
tensor(1244.70312, device='cuda:0', grad_fn=)
tensor(763.75208, device='cuda:0', grad_fn=)
tensor(787.21594, device='cuda:0', grad_fn=)
tensor(20193.73828, device='cuda:0', grad_fn=)
tensor(1710.63989, device='cuda:0', grad_fn=)
tensor(266827.34375, device='cuda:0', grad_fn=)
tensor(2827.42188, device='cuda:0', grad_fn=)
tensor(93085.09375, device='cuda:0', grad_fn=)
tensor(3639.37866, device='cuda:0', grad_fn=)
tensor(92241.87500, device='cuda:0', grad_fn=)
tensor(4282.84180, device='cuda:0', grad_fn=)
tensor(408516.68750, device='cuda:0', grad_fn=)
The text was updated successfully, but these errors were encountered: